
Welcome to the website of the Chair of Physical Chemistry V: Theory and Machine Learning.
We use machine learning (ML) to understand and predict chemical phenomena, such as the nature of complex reaction networks or the properties of new molecules and materials. A major driver of our work is the desire to build accurate, data-efficient models which do not require enourmous reference datasets for training. This is because we want to be able to apply our methods to any problem of chemical interest, not just to those problems for which "big data" happens to be available. To achieve this, we aim to incorporate as much physics as possible into our methods, e.g. by enforcing size-extensivity or a physical description of long-range interactions. This allows performing accurate chemical simulations at unprecedented scales, e.g. to sample large phase spaces or find new functional molecules in chemical space.
The second focus of the group lies in electronic structure theory. Here we are interested in the intersection of wavefunction and density functional methods, with the goal of developing robust and accurate methods that can overcome the large computational costs of the former and the self-interaction problems of the latter. We are also keen on combining ML and electronic structure theory, closing the loop to data-efficient, physics-based ML. A recent example of this is our work on non-local ML-based density functional theory.
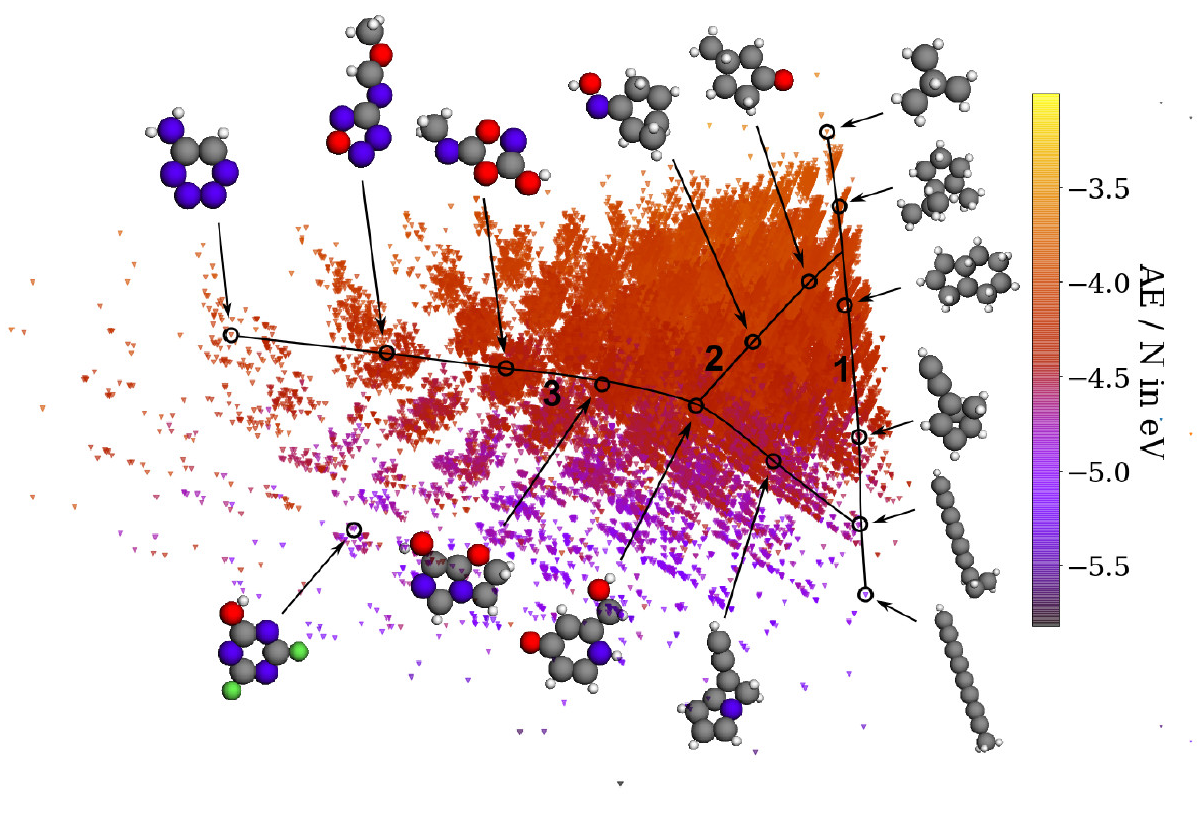
A map of organic molecules, generated using unsupervised machine learning.